The need for a great platform to plan, perform, manage, track and report on A/B tests is of critical importance to our customers as they compete to make better, more profitable data-driven decisions. Often referred to as champion-challenger tests in the banking and risk management worlds, the steps, systems and best practices to make this work can be daunting. At Intelligent Results we've done our best to unite these in single platform, PREDIGY. I've listed several of the key features that PREDIGY provides that you really should know about. This is certainly not an exhaustive list but these little details make being data driven much, much easier.
- IR_ControlNumber - PREDIGY creates and maintains a variable called "IR_ControlNumber" for every dataset loaded into it. This random variable is created at both design-time and run-time allowing it to be used as the basis for strategy splits. IR_ControlNumber is a single numeric variable between 0 and 999 randomly assigned to every account. While each account's number is completely random it can be consistently generated for the same record meaning that the same record will always get the same IR_ControlNumber. This makes this variable ideal for champion-challenger tests over a single campaign or for extended duration tests.
- Segment Name and Strategy Code - Each end node or leaf of the strategy tree has both a Segment Name and a Strategy Code.
a. The Segment Name is a unique identifier for that group of accounts. PREDIGY enforces that these Segment Names are unique which means that at scoring time each account will fall into one and only one segment and that the Segment Name for each account will be logged to the IR Report database. Examples below include "Low Value Control Segment" and "High Value Dialing Segment".
b. The Strategy Code is a non-unique code or score that the strategy will assign to each record at scoring time. You can see below that two of the segments both use "BAU" as the Strategy Code. This means that downstream systems that take action off of this "BAU" code will not know whether the account came from the high or low value segment. Both the Segment Name and Strategy Code for a given account are always available for reporting out of IR Report.
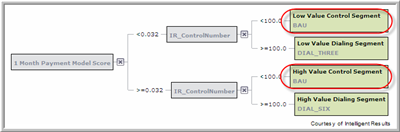
- IR Report – IR Report is designed to capture and report on operational PREDIGY scoring and decisioning applications. Basically, the way it works is that whenever accounts are scored on the IR Production Engine a series of files are written which include all of the input and output values for every record processed. This data is automatically loaded into the IR Report database. A loading program called IRReportDBLoader.exe manages this process, including schema generation, loading and marking files that have already been loaded. Reports can then be run against this IR Report database using PREDIGY’s embedded Crystal Reports or any other query and reporting tools. In general 3 types of reports can be created from IR Report: IT reports focused on the things IT cares about like processing speed, errors, etc...; Statistical reports monitoring input and output variable and score distributions to guard against drift and untimely model aging; and finally, Strategy reports allowing for A/B testing and measurement of the effectiveness of strategies. For these Strategy reports to be complete it is necessary to append outcome data to the database once that data becomes available. What will already be logged into IR Report will include which Segment Name (unique leaf node) and Strategy Code (action that operations should have taken, potentially common to multiple segments) each account fell into on every scoring run. It’s also important to remember that any other account level information provided or generated at scoring time can also be logged into the IR Report database; such as the accounts score on one or more models or strategies (regardless of whether or not they a re used in the current strategy), balance, state, etc...
- Formulas and Actions – The formulas and actions created in design time provide users a simple yet powerful way to estimate and simulate how accounts will flow through their run-time application and what that will mean to their business. In the example below we have calculated several simple measures based on the historic performance of the accounts already loaded into PREDIGY. These include the number and percentage of accounts, the percentage of good and bad accounts and the sum of payments from those accounts in a 30 day period. These formulas and any others that the user wants are easily applied to each node in the decision tree and are updated instantly as the user changes their business rules, models, split points, etc... The Actions and their included formulas combine both calculated measures from the existing records with additional information supplied by the business user allowing you to create estimates, simulations and business cases directly in-line with your decision tree. For example, when we calculate the cost of the "ACTION: Demand Letter" (actions are circled in purple) the formula includes a user defined constant for each letter that will have to be sent for each account. Creating Formulas and Actions is very simple and both can be used throughout the decision tree for data analysis and predicting future business results. Formulas and Actions are purely informational and do not have any impact on the operational scoring process regardless of which actions or formulas you put on various end-nodes or leaves.To affect a downstream system's process you should use Strategy Codes as mentioned above.
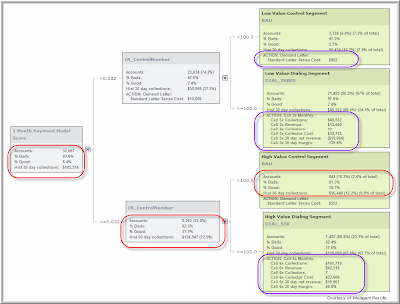
I'm sure there are things I've left out of this write-up but it should give you a starting point to investigate using PREDIGY to manage a larger part of the Modeling, Decisioning, Scoring, Tracking, Reporting and Improving life-cycle. As you read this please feel free to comment and because I've skimmed over several sections like deploying and configuring your application (IRX file), the IR Production Engine and it's web-services and batch options, IR Report, etc... please don't hesitate to send me an email.